Data science has emerged as a transformative force across various sectors, enabling organizations to harness the power of data to drive decision-making, optimize processes, and create innovative solutions. This interdisciplinary field combines statistical analysis, computational techniques, and domain expertise to extract valuable insights from complex data sets. This article explores the fundamentals of data science, its applications, and its impact on various industries.
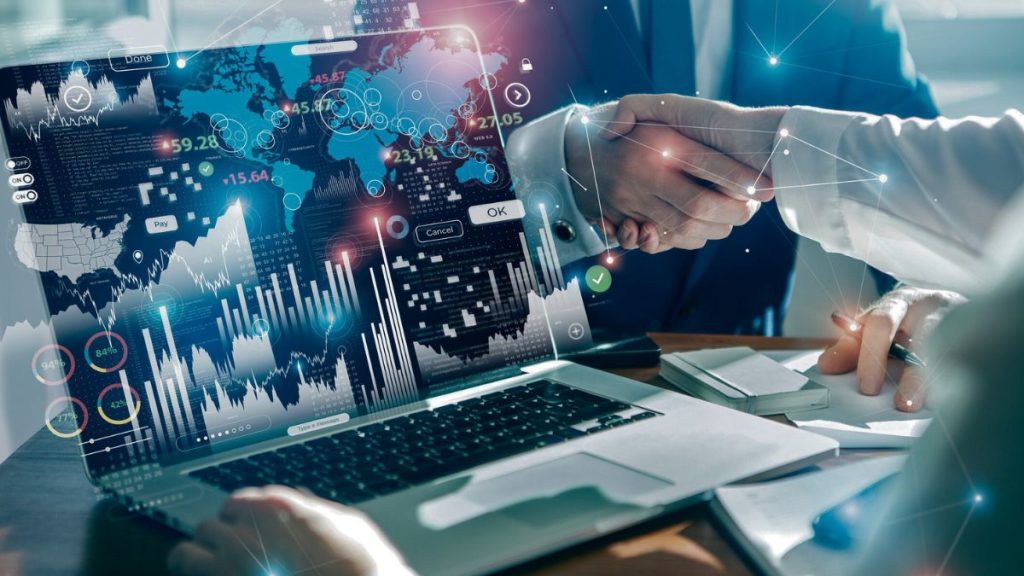
Understanding Data Science
- Definition and Scope:
- What is Data Science?: Data science is the study of extracting meaningful insights from data through various techniques, including statistical analysis, machine learning, and data visualization. It involves collecting, cleaning, analyzing, and interpreting large volumes of data to uncover patterns and inform decision-making.
- Interdisciplinary Nature: Data science integrates knowledge from multiple disciplines, including statistics, computer science, and domain-specific expertise. This interdisciplinary approach enables data scientists to tackle complex problems and develop innovative solutions.
- Core Components:
- Data Collection: The first step in data science is gathering relevant data from various sources, including databases, APIs, and external datasets. Effective data collection ensures that the data is accurate, comprehensive, and representative of the problem being studied.
- Data Cleaning and Preparation: Raw data often contains inconsistencies, missing values, and errors. Data cleaning involves preprocessing and transforming data to ensure its quality and usability. This step is crucial for accurate analysis and modeling.
- Data Analysis and Modeling: Data analysis involves applying statistical methods and machine learning algorithms to identify patterns, trends, and relationships within the data. This step includes exploratory data analysis (EDA), feature engineering, and model training.
- Data Visualization: Communicating insights effectively requires visualizing data in a clear and meaningful way. Data visualization tools and techniques, such as charts, graphs, and dashboards, help present findings and facilitate decision-making.
Applications of Data Science
- Healthcare:
- Predictive Analytics: Data science is used to predict patient outcomes, optimize treatment plans, and identify risk factors. Predictive models can forecast disease outbreaks, personalize medical treatments, and improve patient care.
- Medical Imaging: Machine learning algorithms analyze medical images to detect anomalies, such as tumors or fractures. This technology enhances diagnostic accuracy and supports radiologists in their decision-making process.
- Finance:
- Fraud Detection: Data science helps identify fraudulent activities by analyzing transaction patterns and detecting anomalies. Machine learning models can flag suspicious transactions and prevent financial fraud.
- Algorithmic Trading: Financial institutions use data science to develop trading algorithms that analyze market data and execute trades at high speeds. These algorithms can optimize trading strategies and improve investment returns.
- Retail:
- Customer Segmentation: Data science enables retailers to segment customers based on purchasing behavior, preferences, and demographics. This segmentation allows for targeted marketing campaigns and personalized shopping experiences.
- Inventory Management: Predictive analytics helps retailers forecast demand, optimize inventory levels, and reduce stockouts or overstock situations. This leads to improved supply chain efficiency and cost savings.
- Transportation and Logistics:
- Route Optimization: Data science is used to optimize delivery routes and reduce transportation costs. Machine learning algorithms analyze traffic patterns, weather conditions, and historical data to determine the most efficient routes.
- Predictive Maintenance: Data from sensors and equipment can be analyzed to predict when maintenance is needed, reducing downtime and extending the lifespan of machinery.
- Social Media and Marketing:
- Sentiment Analysis: Data science tools analyze social media posts, reviews, and comments to gauge public sentiment and opinions. This information helps businesses understand customer perceptions and tailor their marketing strategies.
- Recommendation Systems: E-commerce platforms use recommendation algorithms to suggest products based on user preferences and behavior. These systems enhance the shopping experience and increase sales.
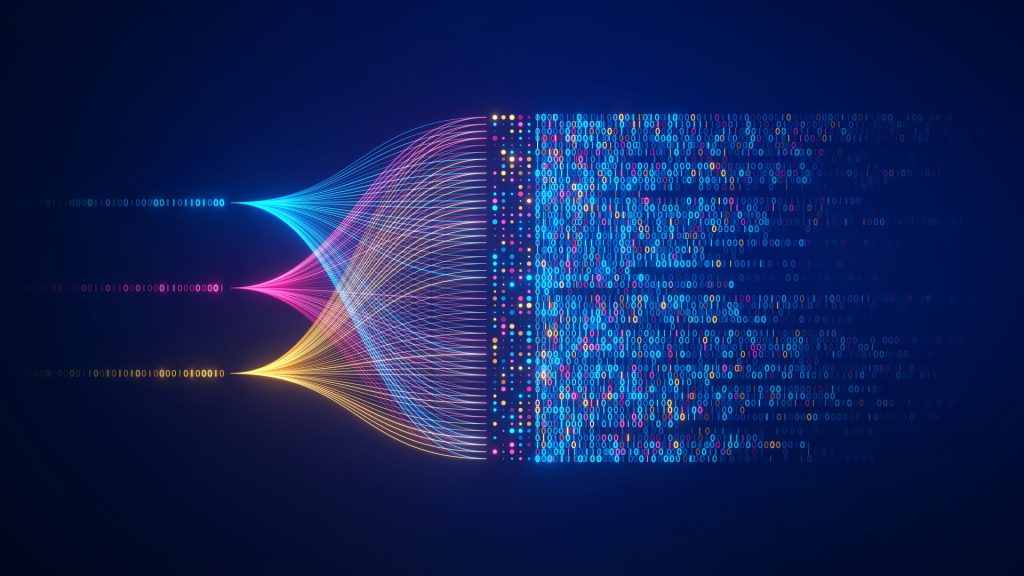
The Future of Data Science
- Advancements in Technology:
- Artificial Intelligence (AI) and Machine Learning: AI and machine learning are integral to data science, enabling advanced analytics and automation. Future developments in these areas will drive innovation and enhance data analysis capabilities.
- Big Data Technologies: The increasing volume, velocity, and variety of data require advanced technologies for processing and analysis. Big data tools and frameworks, such as Apache Hadoop and Spark, will continue to evolve and handle large-scale data challenges.
- Ethics and Privacy:
- Data Privacy: As data collection and analysis become more prevalent, ensuring the privacy and security of personal data is critical. Data scientists must adhere to ethical guidelines and comply with regulations, such as the General Data Protection Regulation (GDPR).
- Bias and Fairness: Data science models can inadvertently perpetuate biases present in the data. Addressing these biases and ensuring fairness in algorithms is essential for building equitable and inclusive systems.
- Interdisciplinary Collaboration:
- Domain Expertise: Successful data science projects require collaboration between data scientists and domain experts. Combining technical skills with industry knowledge ensures that insights are relevant and actionable.
- Cross-Industry Applications: Data science will continue to impact various industries, from agriculture to education. Interdisciplinary approaches and innovative applications will drive progress and address complex challenges.
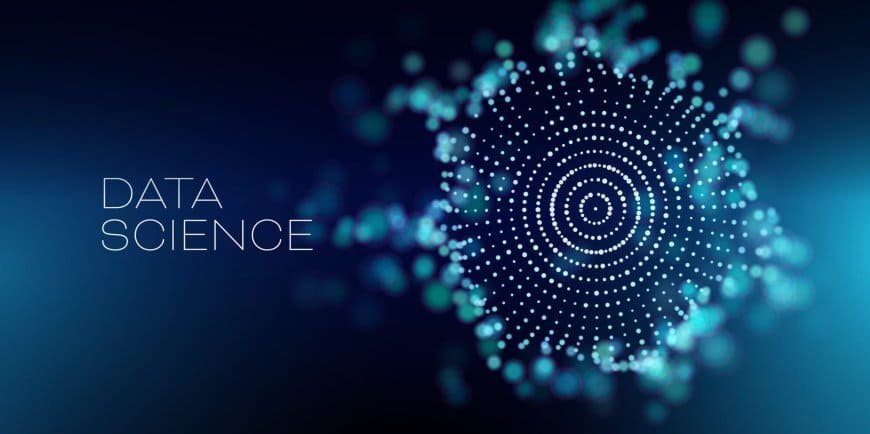
Conclusion
Data science has become a cornerstone of modern decision-making and innovation, offering valuable insights and solutions across a wide range of industries. By leveraging advanced analytics, machine learning, and data visualization, organizations can harness the power of data to drive growth, improve efficiency, and enhance customer experiences. As technology continues to advance and data volumes increase, the field of data science will play an increasingly vital role in shaping the future of business and society. Embracing data science and its potential will be key to staying competitive and unlocking new opportunities in the ever-evolving digital landscape.