Artificial Intelligence (AI) has made significant strides across various sectors, and one of the most promising applications is in drug discovery. AI-driven drug discovery is transforming how new medications are developed, offering the potential to accelerate the process, reduce costs, and improve the efficacy of drugs. This article explores how AI is reshaping drug discovery, its benefits, challenges, and the future of this innovative approach.
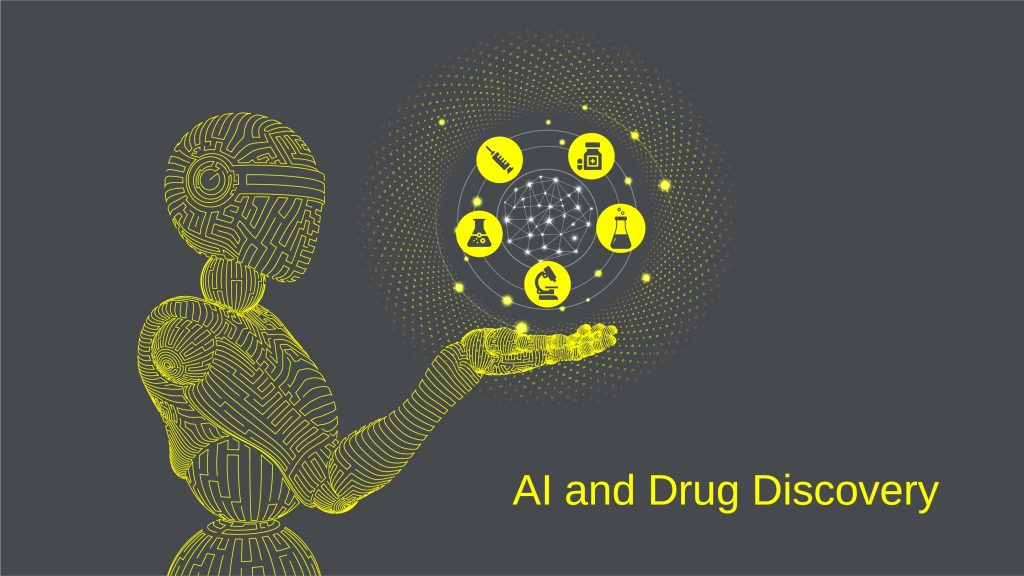
Understanding AI-Driven Drug Discovery
- What is AI-Driven Drug Discovery?
- Definition: AI-driven drug discovery involves using artificial intelligence and machine learning techniques to identify, develop, and optimize new pharmaceuticals. By analyzing vast amounts of biological and chemical data, AI models can predict how different compounds will interact with biological targets, thus facilitating the identification of potential drug candidates.
- Key Technologies: The core technologies used in AI-driven drug discovery include machine learning algorithms, natural language processing (NLP), and data analytics. These technologies help in analyzing complex datasets, predicting molecular interactions, and identifying patterns that may not be evident through traditional methods.
- How AI Enhances Drug Discovery:
- Data Integration and Analysis: AI can process and integrate large datasets from various sources, including genetic, proteomic, and chemical data. This comprehensive analysis helps identify potential drug targets and biomarkers more efficiently than traditional methods.
- Predictive Modeling: AI models can predict how different compounds will affect biological systems. By simulating molecular interactions and drug responses, AI can suggest which compounds are most likely to be effective and safe, streamlining the drug development process.
Applications of AI in Drug Discovery
- Target Identification and Validation:
- Biomarker Discovery: AI can analyze genomic and proteomic data to identify biomarkers associated with specific diseases. These biomarkers can serve as targets for drug development, enabling the creation of more targeted and effective treatments.
- Protein-Protein Interactions: AI models can predict interactions between proteins, which is crucial for understanding disease mechanisms and identifying potential drug targets. This insight helps in designing drugs that specifically modulate these interactions.
- Compound Screening and Optimization:
- Virtual Screening: AI-driven virtual screening involves using machine learning algorithms to evaluate large libraries of chemical compounds. By predicting which compounds are likely to interact with a target, AI can prioritize candidates for experimental testing, reducing the number of compounds that need to be tested in the lab.
- De Novo Drug Design: AI can generate new drug-like molecules through de novo design, optimizing chemical structures to improve efficacy and reduce side effects. These AI-generated compounds can be tested experimentally to identify promising drug candidates.
- Preclinical and Clinical Trials:
- Predicting Drug Toxicity: AI models can predict potential toxicity and adverse effects of drug candidates by analyzing historical data and simulating drug interactions. This predictive capability helps in identifying and mitigating safety risks early in the drug development process.
- Patient Stratification: AI can analyze patient data to identify subgroups that are more likely to respond to specific treatments. This approach, known as precision medicine, enhances the design of clinical trials and improves the likelihood of successful outcomes.
Benefits of AI-Driven Drug Discovery
- Accelerated Drug Development:
- Reduced Timeframes: AI can significantly shorten the time required for drug discovery by streamlining the identification of drug candidates and optimizing their development. This acceleration is crucial for addressing urgent medical needs and responding to emerging health crises.
- Efficient Resource Allocation: By prioritizing the most promising candidates and reducing the number of experimental tests needed, AI helps allocate resources more efficiently. This efficiency leads to cost savings and faster time-to-market for new drugs.
- Increased Accuracy and Success Rates:
- Improved Predictions: AI models provide more accurate predictions of drug interactions and responses, leading to higher success rates in drug development. This accuracy enhances the likelihood of discovering effective and safe medications.
- Novel Drug Candidates: AI’s ability to generate new compounds and identify novel drug targets expands the pool of potential drug candidates. This innovation can lead to the development of drugs for previously untreatable conditions.
- Personalized Medicine:
- Tailored Treatments: AI-driven drug discovery enables the development of personalized treatments based on individual genetic and molecular profiles. This approach enhances treatment efficacy and reduces the risk of adverse effects by tailoring medications to specific patient needs.
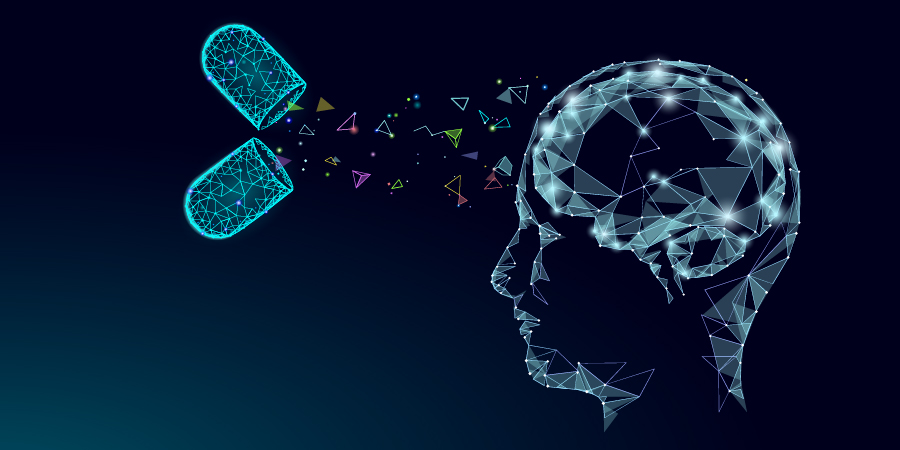
Challenges and Considerations
- Data Quality and Availability:
- Data Integration: AI-driven drug discovery relies on high-quality, diverse datasets. Integrating and standardizing data from various sources can be challenging and may affect the accuracy of AI models.
- Data Privacy: Handling sensitive patient data requires robust privacy measures and compliance with regulations. Ensuring data security and ethical use of information is crucial for maintaining trust and integrity in AI-driven drug discovery.
- Model Limitations:
- Algorithmic Bias: AI models can inherit biases present in training data, leading to biased predictions and potential disparities in drug development. Addressing these biases and ensuring fairness in AI-driven drug discovery is essential for achieving equitable outcomes.
- Interpretability: Many AI models, particularly deep learning algorithms, operate as “black boxes,” making it difficult to interpret their decision-making processes. Improving model interpretability and understanding how AI arrives at conclusions is important for validating and trusting AI-generated insights.
- Regulatory and Ethical Issues:
- Regulatory Approval: AI-driven drug discovery must navigate complex regulatory requirements for drug approval. Ensuring that AI-generated drug candidates meet regulatory standards and demonstrate safety and efficacy is a critical consideration.
- Ethical Implications: The use of AI in drug discovery raises ethical questions related to the responsible use of technology and the impact on patients. Addressing these ethical concerns and ensuring that AI is used for the benefit of society is essential.
The Future of AI-Driven Drug Discovery
- Advancements in AI Technologies:
- Enhanced Algorithms: Future developments in AI technologies will likely lead to more sophisticated algorithms that improve the accuracy and efficiency of drug discovery. Continued research and innovation will push the boundaries of what AI can achieve in this field.
- Integration with Other Technologies: Combining AI with other emerging technologies, such as genomics and bioinformatics, will further enhance drug discovery capabilities. Integrated approaches will provide a more comprehensive understanding of diseases and drug interactions.
- Collaborative Efforts:
- Partnerships and Collaboration: Collaboration between AI researchers, pharmaceutical companies, and academic institutions will be crucial for advancing AI-driven drug discovery. Partnerships will facilitate knowledge sharing, resource pooling, and the development of innovative solutions.
- Ethical and Responsible Development:
- Regulatory Frameworks: Developing robust regulatory frameworks and ethical guidelines for AI-driven drug discovery will ensure responsible and effective use of technology. Policymakers, researchers, and industry stakeholders must work together to address regulatory and ethical challenges.
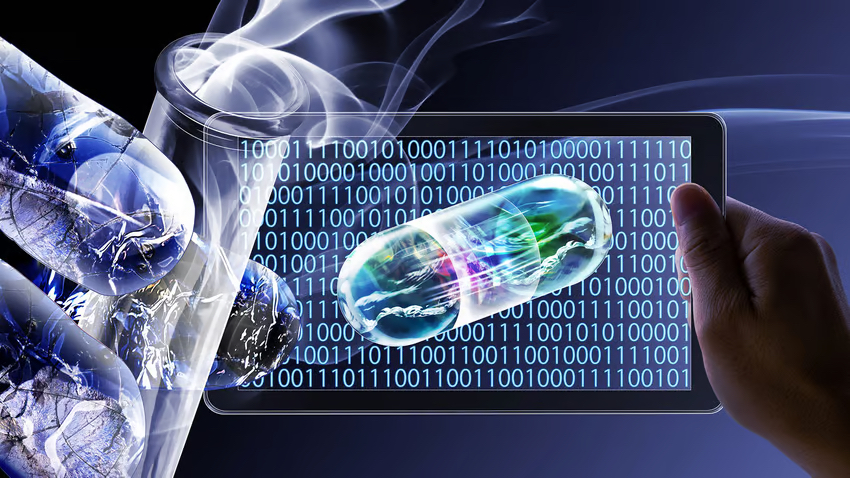
Conclusion
AI-driven drug discovery is transforming the pharmaceutical industry by accelerating the development of new medications, improving accuracy, and enabling personalized treatments. While challenges related to data quality, model limitations, and regulatory issues remain, the potential benefits of AI in drug discovery are significant. As AI technologies continue to advance, they will play an increasingly important role in shaping the future of medicine and addressing global health challenges. Embracing the potential of AI while addressing its challenges will be key to unlocking the full promise of this revolutionary approach to drug discovery.