In today’s data-driven world, organizations are constantly seeking ways to harness vast amounts of data to gain actionable insights. Traditional analytics approaches often struggle to keep pace with the growing complexity and volume of data. This is where augmented analytics comes into play, combining the power of artificial intelligence (AI) with advanced analytics to enhance data insights and decision-making processes. This article explores the concept of augmented analytics, its benefits, and its impact on business intelligence.
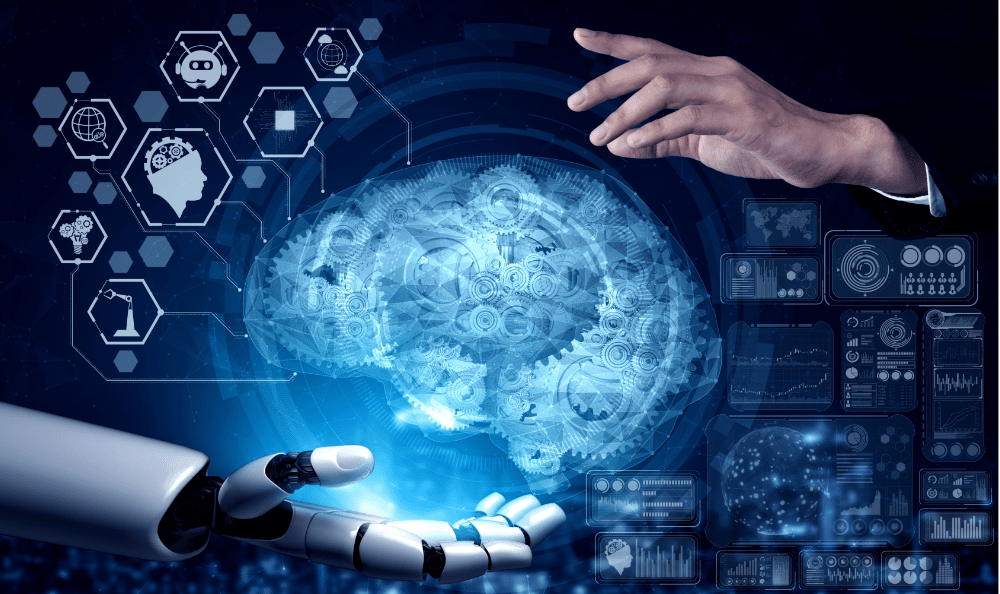
What is Augmented Analytics?
- Definition:
- Augmented Analytics: Augmented analytics refers to the use of AI, machine learning, and natural language processing (NLP) to automate data preparation, insight discovery, and sharing. It enhances traditional analytics by providing deeper, more accurate insights and making data analysis more accessible to non-experts.
- Automation: By automating routine tasks such as data cleansing, integration, and analysis, augmented analytics allows data professionals to focus on higher-level strategic work, while also enabling business users to independently explore data and gain insights.
- Components of Augmented Analytics:
- AI and Machine Learning: These technologies help in identifying patterns, predicting outcomes, and generating insights without requiring manual intervention.
- Natural Language Processing (NLP): NLP enables users to interact with data using conversational language, making data analysis more intuitive and accessible.
- Automated Data Preparation: This involves the automated cleansing, transformation, and integration of data from various sources, ensuring that the data is ready for analysis.
The Benefits of Augmented Analytics
- Enhanced Data Insights:
- Deep Learning: AI-driven analytics can uncover hidden patterns and correlations within data that may be missed by traditional methods. This leads to more accurate and comprehensive insights.
- Predictive Analytics: Augmented analytics enables predictive modeling, allowing organizations to anticipate future trends and make proactive decisions.
- Improved Decision-Making:
- Data-Driven Culture: By making advanced analytics more accessible, augmented analytics promotes a data-driven culture within organizations. Decision-makers at all levels can leverage data insights to inform their strategies.
- Real-Time Insights: Augmented analytics can provide real-time data insights, enabling faster and more informed decision-making in dynamic business environments.
- Increased Productivity:
- Automation of Routine Tasks: Automating tasks such as data preparation and analysis frees up time for data professionals, allowing them to focus on more complex and strategic work.
- Empowering Non-Technical Users: Augmented analytics tools are designed to be user-friendly, enabling non-technical users to perform sophisticated data analysis without the need for specialized skills.
- Scalability and Flexibility:
- Handling Large Data Sets: Augmented analytics tools are capable of processing and analyzing large volumes of data quickly and efficiently, making them ideal for organizations dealing with big data.
- Adaptability: These tools can be adapted to various industries and business contexts, providing tailored insights that meet specific organizational needs.
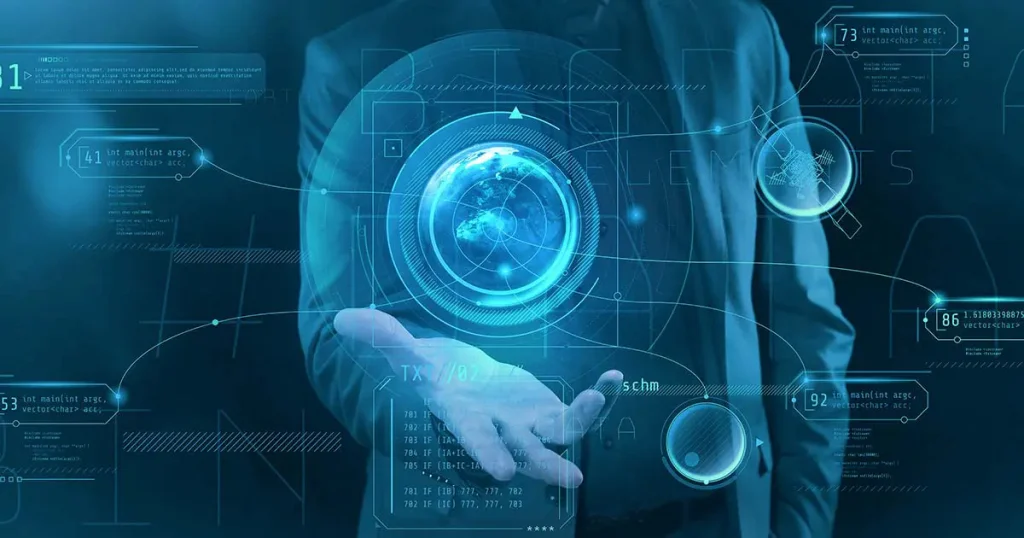
Impact on Business Intelligence
- Transforming Traditional BI:
- Augmented BI: Augmented analytics is revolutionizing traditional business intelligence (BI) by enhancing the capabilities of BI tools with AI and machine learning. This shift is leading to more sophisticated and automated insights.
- User Empowerment: Traditional BI often required the involvement of data analysts or IT professionals. Augmented analytics democratizes data access, empowering business users to generate their own insights.
- Personalized Insights:
- Customized Analytics: Augmented analytics can deliver personalized insights based on individual user preferences, roles, and data access levels. This personalization enhances the relevance and usefulness of the insights.
- Contextual Understanding: AI-driven analytics tools can provide context-aware insights, taking into account the specific business environment, industry trends, and historical data.
- Integration with Existing Systems:
- Seamless Integration: Augmented analytics can be integrated with existing BI and data management systems, enhancing their capabilities without the need for a complete overhaul.
- Unified Data Ecosystem: By integrating augmented analytics with other data tools, organizations can create a unified data ecosystem that supports more effective decision-making.
Challenges and Considerations
- Data Quality and Management:
- Ensuring Data Integrity: The effectiveness of augmented analytics depends on the quality of the data being analyzed. Organizations must prioritize data governance and management to ensure accuracy and reliability.
- Data Privacy: With the increasing use of AI and data analytics, organizations must also address concerns related to data privacy and compliance with regulations such as GDPR.
- User Adoption:
- Training and Education: While augmented analytics tools are designed to be user-friendly, organizations may still need to invest in training and education to ensure that users can effectively leverage these tools.
- Change Management: Implementing augmented analytics requires a cultural shift towards data-driven decision-making. Organizations must manage this change to ensure successful adoption.
- Ethical Considerations:
- AI Bias: The use of AI in analytics raises concerns about potential biases in algorithms, which can lead to skewed insights. Organizations must be vigilant in monitoring and mitigating AI bias.
- Transparency: Ensuring transparency in how AI-driven insights are generated is crucial for building trust among users and stakeholders.
The Future of Augmented Analytics
- Continued Innovation:
- AI Advancements: As AI and machine learning technologies continue to evolve, augmented analytics will become even more powerful, offering deeper insights and more sophisticated predictive capabilities.
- Integration with Emerging Technologies: The future of augmented analytics may involve integration with emerging technologies such as IoT, blockchain, and edge computing, further enhancing its potential.
- Wider Adoption:
- Industry Adoption: Augmented analytics is expected to see widespread adoption across various industries, from finance and healthcare to retail and manufacturing, as organizations seek to stay competitive in a data-driven world.
- SME Accessibility: As augmented analytics tools become more affordable and accessible, small and medium-sized enterprises (SMEs) will also benefit from these advanced analytics capabilities.
- Ethical AI Development:
- Focus on Fairness: The development of fair and unbiased AI algorithms will be a key focus, ensuring that augmented analytics delivers equitable and accurate insights for all users.
- Regulatory Compliance: As regulations around AI and data analytics evolve, organizations will need to ensure that their use of augmented analytics complies with legal and ethical standards.
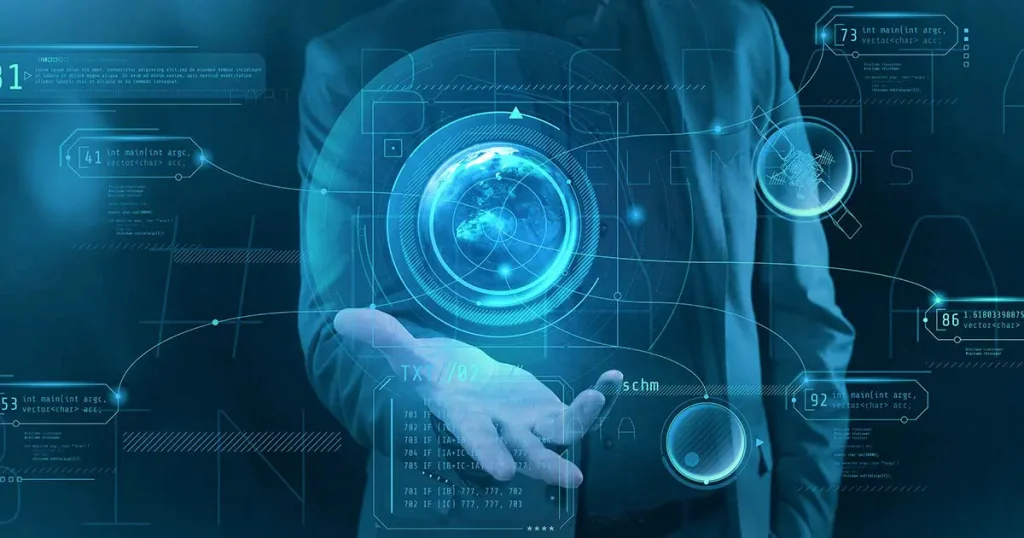
Conclusion
Augmented analytics represents a significant leap forward in the field of data analytics, combining the power of AI with advanced analytics to deliver deeper insights and more informed decision-making. As organizations continue to navigate the complexities of a data-rich world, augmented analytics offers a powerful tool to enhance productivity, drive innovation, and create a more data-driven culture. By addressing challenges related to data quality, user adoption, and ethical considerations, businesses can fully harness the potential of augmented analytics to stay ahead in an increasingly competitive landscape.